Editor's Note: Enjoy this blog from the Custora archive, acquired by Amperity in November 2019.
To successfully communicate with the customer, marketers need to be able to generate insights with enough lead time to actually use them.
We've talked a lot about customer segmentation lately because strategically designed, well-made segments are the key to giving each customer the red carpet treatment while minimizing cost and time investment. In this post, we reveal all of the ingredients you need to successfully cook up a winning segmentation strategy.
Improving marketing communications begins with asking mission-critical customer questions — and answering them in a timely manner. That’s the most foolproof way to retain customers and keep them from switching to another brand. Formulating the questions is the easy part; we have a few favorite customer-centric metrics that we think every retail marketer should be monitoring, including customer lifetime value (CLV), customer equity, and customer retention.
But quickly measuring and utilizing those key performance indicators (KPIs) is another story. Reaching a “nirvana” state of agile, coordinated, and iterative marketing communications requires a set of six critical capabilities. These capabilities are divided into two parts: generating customer insights, and making those insights easy to use across the organization. Let’s take a closer look at all six capabilities now.
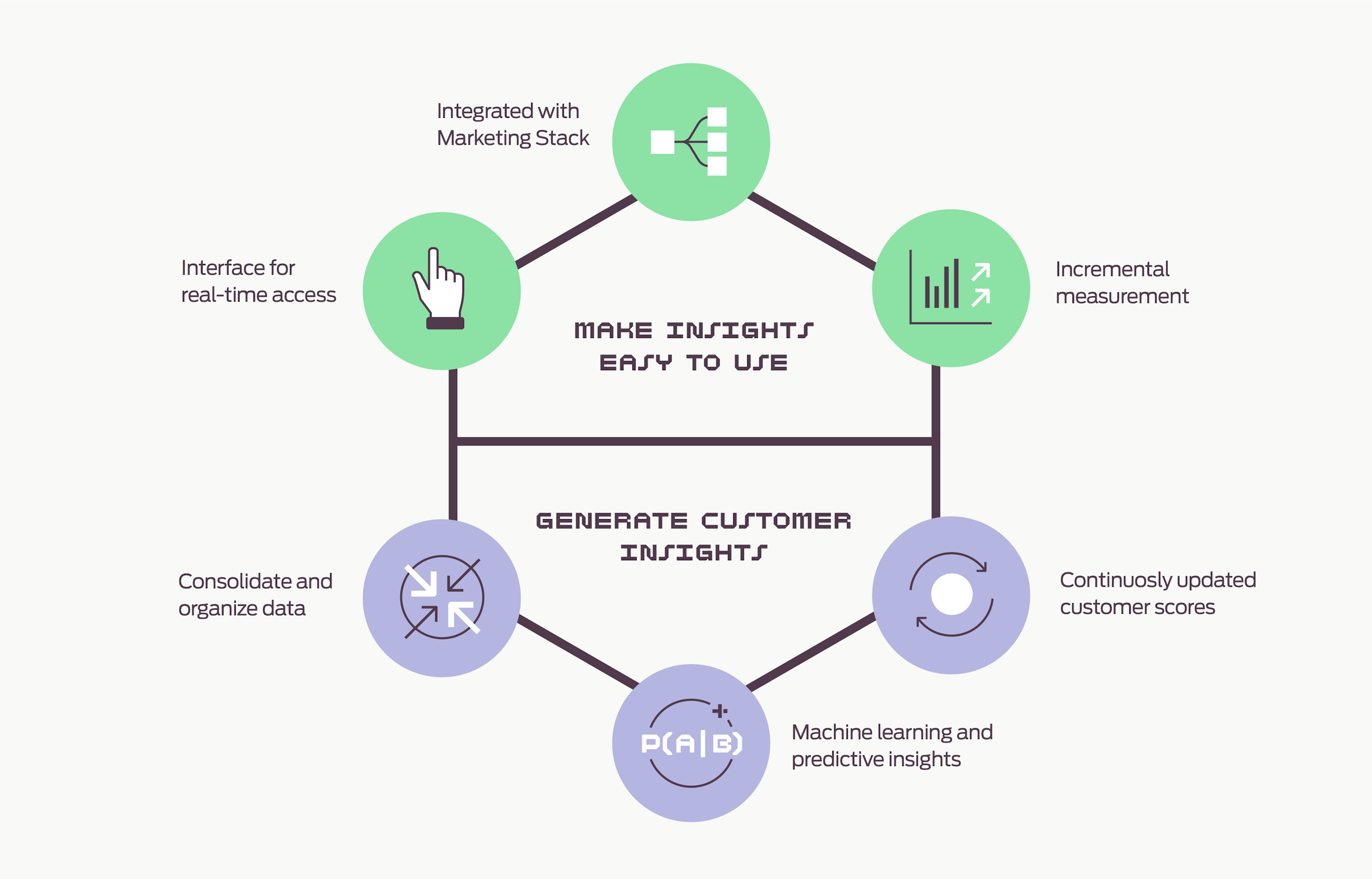
Generating Customer Insights
Consolidate data: We can’t analyze customers before we gather and consolidate all of our existing customer data, which can be trickier than it sounds. For retailers who work across channels, connecting the dots to understand that the Larry who made an in-store purchase in 2015 is the same Larry who made an online order a year later requires some finagling (online-only retailers, consider yourselves lucky).
Sometimes, loyalty programs can help retailers figure out that Larry and Larry are, in fact, the same Larry, but in the absence of such programs, “fuzzy matching” is necessary. Fuzzy matching isn’t nearly as cute as it sounds — it means scouring purchase records for names, emails, and home addresses, and then layering in other aspects of customer behavior like browsed products, opened emails, and demographic attributes to try to consolidate multiple purchases into a useful customer portrait. Some machine learning platforms can assist with this process.
Machine learning and predictive insights: There’s a big gap between “having a lot of data” and “generating customer insights,” and advanced analytics are the bridge that connects them. Anticipating consumer behavior is the new standard for marketing analytics, and with millions of data points, those predictions require a substantial amount of data science to marry historical data with predictive modeling.
Teams that rely on machine learning to do this are going to have a much easier time of it; machine learning technology can offer insights into critical variables like predicted customer lifetime value ((P)CLV) even when there is limited data available, as well as other information like product affinities, promotional preferences, and engagement preferences.
Making Insights Easy to Use
Updated customer scores: Making data useful means separating the signal from the noise. While layering on predictive insights for each customer can drive tremendous value, we can’t expect marketers to comb through virtually endless piles of data looking for the needle in the haystack. Marketers need to know which of those insights are likely to drive the biggest gains; otherwise, they’ll never be able to turn all of that data into meaningful action.
For example, an advanced analytics team might surface a data point on each customer described as “product category most likely to be purchased this week.” That sounds exciting and actionable — if the company is trying to sell more denim that week, they can identify denim shoppers and target them with a discount email. But that raises the question: should we segment based on product affinities in that manner? Perhaps segmenting coupon clippers for the discount email would be more profitable. Segments alone don’t offer guidance on which segments are useful, which is what prescriptive analytics can do.
Interface for real-time access: Rich customer insights are a prerequisite to any sophisticated strategic analysis, but it’s often far too cumbersome for marketers to gain these insights. Many organizations have Advanced Analytics or CRM teams that are capable of providing these meaningful customer insights, but they usually serve the entire organization — so when marketers need information from them, it means filing a ticket and waiting, sometimes for months.
More often than not, by the time these data insights reach marketing teams, the information is no longer actionable. That’s why marketers need an intuitive, accessible interface that offers real-time insights; this can fundamentally change the way the team leverages data, driving real growth and repeat purchases.
Integration with marketing stack: Marketers should have a simple way to build an audience and push that audience into all their execution tools, but that’s easier said than done. Different execution tools rely on different customer information, creating friction in the “audience pushing” process that often leads marketers to simply rely on email for targeted messaging.
Without integrating insights across the entire marketing stack, marketing teams often fall back on letting each team do their own thing with little to no coordination between them. Teams that are able to find “the customer” across the stack, on the other hand, are able to more rapidly experiment with consistent, cross-channel communication — together.
Incremental measurement: A customer-centric marketing organization needs to be able to measure success on the customer level; channel- or campaign-specific data isn’t enough. This requires that marketers be able to access data analysis without reaching out to the CRM or Advanced Analytics team for support.
When marketers can run tests and analyze the results independently and in real time, they find the right tactics more quickly and efficiently — and real, sustainable growth begins with pinpointing the right tactics.
Together, these six points form a comprehensive Customer Analytics Platform, which should sit at the center of your marketing stack. Once those six core capabilities have been established, your retail organization is all set to start marketing at the customer level and consistently tantalizing customers to come back for repeat purchases.